Build data pipelines, tests, and docs with Jupyter notebooks
data chimp is a VSCode plugin that turns Jupyter notebooks into a full-blown data and ML workspace. We're currently in open beta with some features still in closed beta.
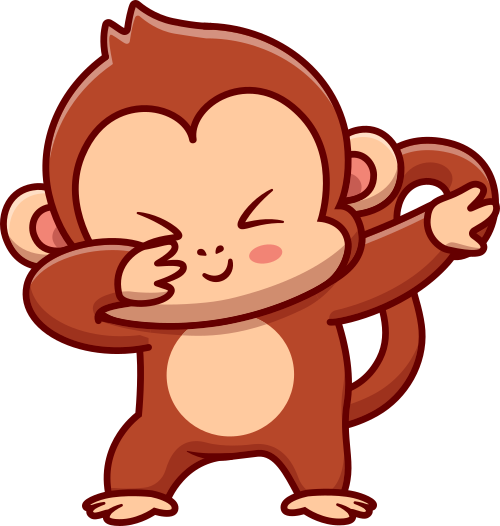
Go from notebook cells to testable functions to pipelines in a few clicks
data chimp analyzes your code to turn a notebook cell into a isolated, unit-testable function. Then, it gives you a visual pipeline builder turn those functions into a pipeline that's run on your own infrastructure. data chimp doesn't need access to your code or database keys.
Validate your data and ML models with automatically-run, pre-built checks or create your own with a Jupyter notebook
data chimp automatically runs configurable data and model checks as you work in your notebook AND as you run your pipelines.
Here, data chimp is automatically showing a set of rows that violate a data assertion we made about petal_length.
Get and create data docs within your Jupyter notebooks
Isn't it insane that notebooks aren't used for data docs? Flag bad columns for your team or integrate with dbt docs.
Automate the boring parts of EDA
data chimp automatically generates configurable, contextual visualizations and statistics to help you explore your data faster. You can even send the code that generated a visualization back into a cell for further iteration. Think pandas profiling, but better.